Microseismic event monitoring has become a primary diagnostic tool of the hydraulic fracturing of the unconventional oil and gas reservoirs. The goal is to provide useful microseismic information, such as when and where did the event occur, and what type of event was it? Engineers can make better decisions.
The signals in microseismic monitoring that are recorded by surface or near-surface array typically have rather low amplitudes buried in noise and interference require sophisticated method to provide optimal processing. The goal of this project is to develop novel efficient and reliable microseismic monitoring schemes, such as event detection algorithms, location algorithms, and hopefully moment tensor inversion. From an application’s point of view, the newly developed algorithms and some necessary simulations are planned to be programmed.
Research Outcomes:
Some of the research outcomes of the project are:
- Sparse-promoting Full Waveform Inversion Based on Online Orthonormal Dictionary Learning
- Microseismic Events Enhancement and Detection in Sensor Arrays
Sparse-promoting Full Waveform Inversion Based on Online Orthonormal Dictionary Learning
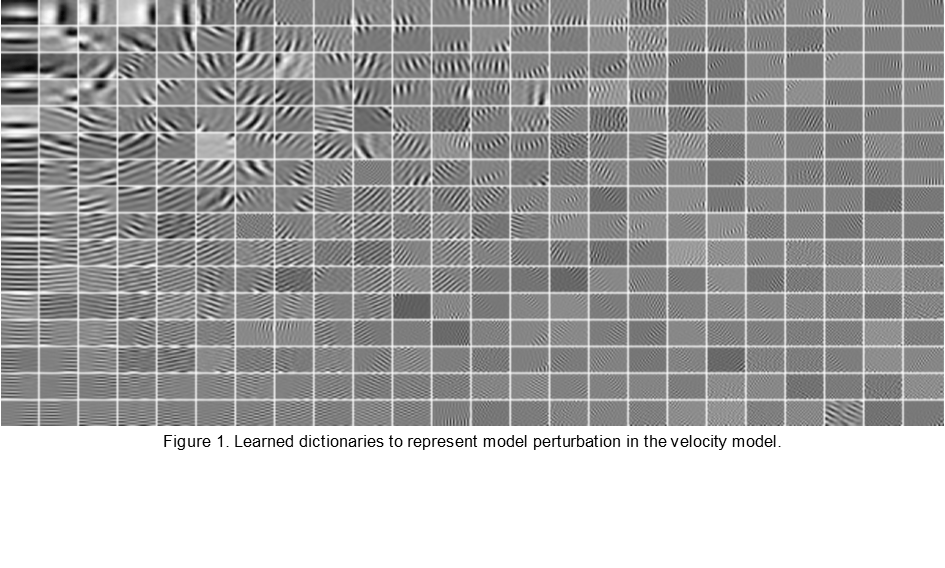
During the latter half of 2015, we proposed a new Full Waveform Inversion (FWI) method based on the dictionary-learning method and submitted two papers. One is accepted by International Conference on Acoustics, Speech and Signal Processing (ICASSP) 2016 in Shanghai, China and another one is pending review in Geophysics.
FWI delivers high-resolution subsurface geophysical models by iteratively minimizing the least-squares misfit between the observed and simulated seismic data. Due to the limited accuracy of the starting model and the inconsistency of the seismic waveform data, the FWI problem is inherently ill-posed, so that regularization techniques are typically applied to obtain better models. FWI is also a computationally expensive problem because modern seismic surveys cover very large areas of interest and collect massive volumes of data. The dimensionality of the problem and the heterogeneity of the medium both stress the need for faster algorithms and sparse regularization techniques to accelerate and improve imaging results.
Our method reaches these goals by developing a compressive sensing approach for the FWI problem, where the sparsity of model perturbations is exploited within learned dictionaries. Dictionaries are updated iteratively to adapt to dynamic model perturbations. Meanwhile, the dictionaries are kept orthonormal in order to maintain the corresponding transform in a fast and compact manner without introducing extra computational overhead to FWI. Such a sparsity regularization on model perturbations enables us to take randomly subsampled data for computation and thus significantly reduce the cost.

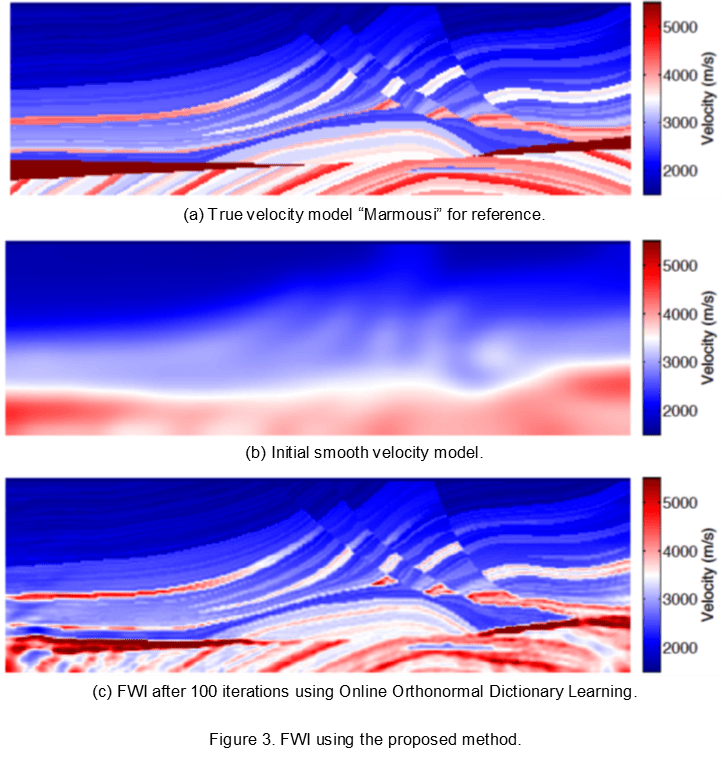
References:
1. L. Zhu, E. Liu, and J. McClellan, “Sparse-promoting full waveform inversion based on online orthonormal dictionary learning,” submitted to Geophysics.
2. L. Zhu, E. Liu, and J. H. McClellan, “Fast online orthonormal dictionary learning for efficient full waveform inversion,” to be presented at IEEE Intl. Conf. on Acoustics, Speech and Signal Processing (ICASSP), Shanghai, China, Mar. 20-25, 2016.
Microseismic Events Enhancement and Detection in Sensor Arrays
Passive microseismic data are commonly buried in noise, which presents a significant challenge in microseismic data analysis and event detection. One of the ongoing topics is to provide efficient and robust algorithms for the microseismic event detection and denoising. Practically, we consider the situation where a sensor array (downhole or on the surface) provides multiple traces that each contain an arrival from the event. We have proposed an autocorrelation-based method that designs a denoising filter in the frequency domain which is then applied to all the traces to make the event detection easier. This approach uses stacking of the autocorrelation functions, which works well because the autocorrelations of all the traces are centered at zero in the lag domain. Thus, it does not suffer the usual drawback of stacking where the time offsets among traces must be known. The performance of the method is studied with respect to the number of traces, the noise level, as well as the spectra of the wavelet and the noise.
The simplicity and robustness of this method is validated with synthetic and field datasets. In Figure 1 (a) to (c), we show the original data (only showing 14 traces out of 200), noisy data after adding Gaussian noise, and the denoised results. The interested reader is referred to the CeGP publications.
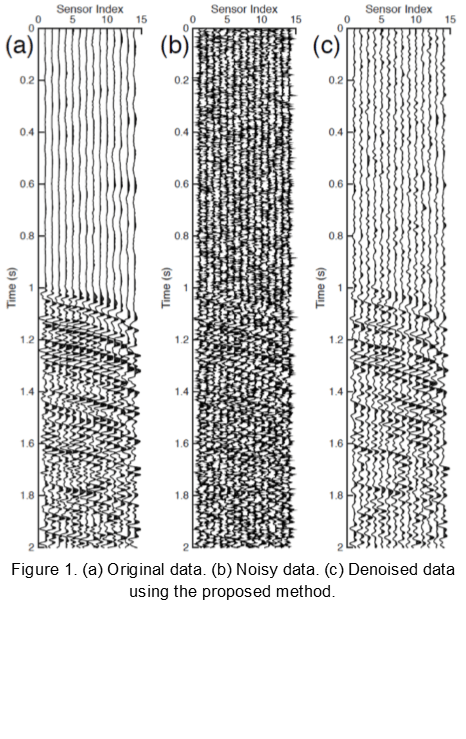
References:
1. E. Liu, L. Zhu, and J. H. McClellan, A. Al-Shuhail, S. I. Kaka, “Microseismic events enhancement in sensor arrays using autocorrelation based filtering,” submitted to the 78th EAGE Annual Conference & Exhibition, Vienna, Austria, May 30-June 2, 2016.
2. L. Zhu, E. Liu, and J. H. McClellan, “”An automatic arrival time picking method based on RANSAC curve fitting,” submitted to 78th EAGE Annual Conference & Exhibition, Vienna, Austria, May 30-June 2, 2016.
3. L. Zhu, E. Liu, and J. H. McClellan, “Full waveform microseismic inversion using differential evolution algorithm,” Proc. IEEE Global Conf. on Signal and Information Processing (GlobalSIP), Orlando, Florida, Dec. 14-16, 2015.
The Team:
Name | Title | Affiliation |
---|---|---|
Dr. Abdullatif Al-Shuhail | Associate Professor | Earth Sciences Department, KFUPM |
Dr. SanLinn Isma’il Kaka | Associate Professor | Earth Sciences Department, KFUPM |
Dr. Azzedine Zerguine | Professor | Electrical Engineering Department, KFUPM |
Dr. Naveed Iqbal | Post-Doctoral Associate | Electrical Engineering Department, KFUPM |
Arbab Latif Azhar Latif | Graduate Student | Department of Electrical Engineering, KFUPM |
Syed Salahuddin Bacha | Graduate Student | School of Department of Electrical Engineering, KFUPM |
Mohammad Umair Mohammad Yaqub | Graduate Student | School of Department of Electrical Engineering, KFUPM |
Ryan Adhi Putra | Graduate Student | School of Department of Earth Science, KFUPM |
Dr. James McClellan | Professor | School of Electrical and Computer Engineering, Georgia Tech |
Dr. Entao Liu | Post-Doctoral Fellow | School of Electrical and Computer Engineering, Georgia Tech |
Lijun Zhu | Graduate Student | School of Electrical and Computer Engineering, Georgia Tech |